Thursday 5PM (ET): The one and only Stephen Wolfram goes deep on AI
Friday 2PM (ET): Disco CEO Evin McMullen explains how to prove you're human in an AI world.
Inside Today's Meteor
- Disrupt: What Happens When ChatBots Escape the Lab?
- Create: The Absurd AI Art of InfiniteYay
- Compress: Vinay Gupta Knows What Amazon Should Do Next
- Tools: Runway's Exciting Text-to-Video Launch
What Happens When ChatBots Escape the Lab?
Welcome to chapter 2 of our recent report about the Meta AI jailbreak. This story keeps growing, and it may be one of the most important and under-discussed developments in AI right now. Probably because no one is going to make any money off it, legally anyway.
Stanford researchers have created a new language model, dubbed Alpaca.cpp, that performs nearly as well as OpenAI's text-davinci-003 model, and it's small enough to run on a phone. They say it cost about $600 to produce.
This is a big deal because it's also a proof that you can right-click-save large language models (LLMs) – like OpenAI's ChatGPT – that took years, hundreds of millions of dollars and massive supercomputers to develop. It won't make a perfect copy, but good enough, like a lossy compressed version of a high definition photo that drops out some of the pixels to make the file size smaller. It's not quite as crisp, but it's legible and easier to put on a Web server.
So how did they pull it off?
LLaMA was created on purpose to be small, in order to facilitate exactly this kind of experimentation. Normally running a large language model (LLM) like OpenAI's text-davinci-003 would be impossible outside of a specialized lab. In that context, bigger is always better, and text-davinci-003 got elite private school level training with 175B parameters.
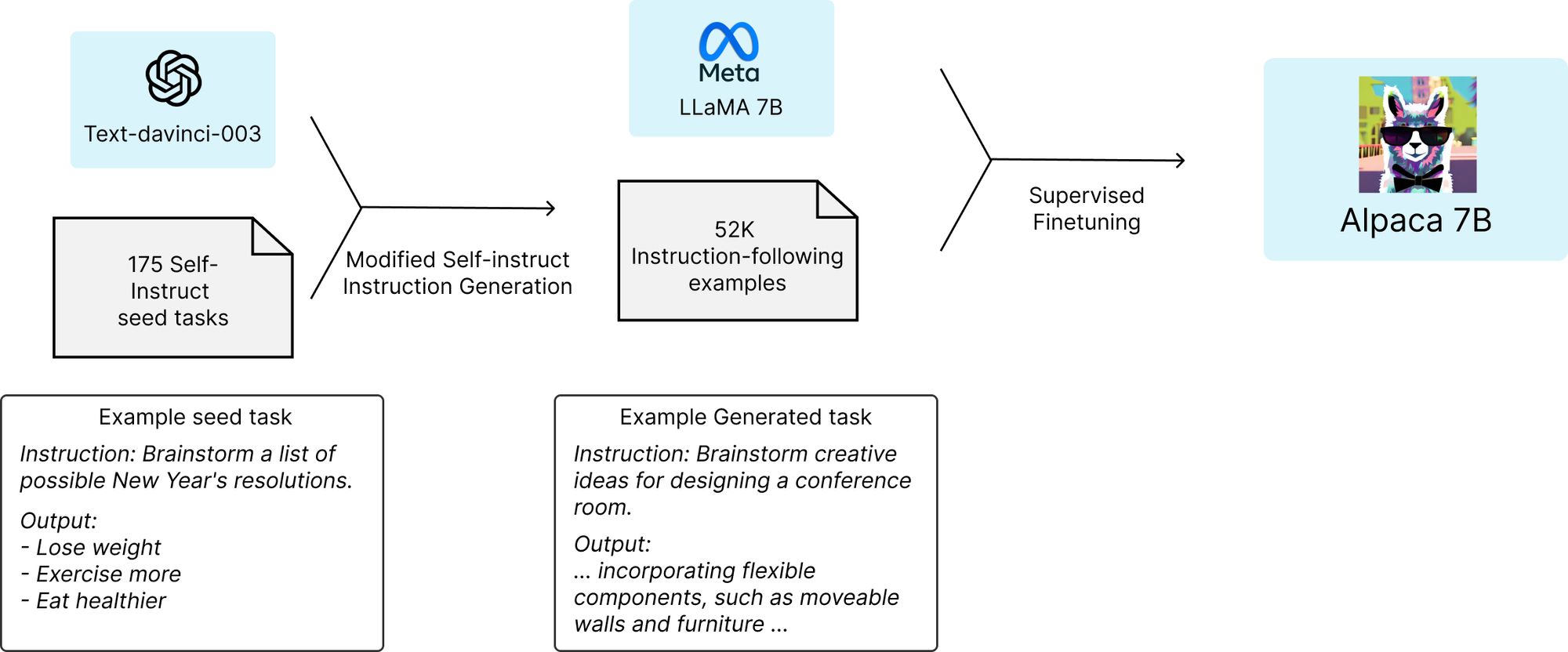
The Stanford team retrained Meta's leaked public school 7B parameter LLaMA model on a small sample of results pulled via the OpenAI's API. Then they basically pointed at what OpenAI's version did and told LLaMA, "Do it like that."
And it did.
"Alpaca shows many behaviors similar to OpenAI’s text-davinci-003, but is also surprisingly small and easy/cheap to reproduce," the authors write, in what could be the understatement of the year.
The release, offered under a noncommercial license only, comes as the debate over closed and open research in AI is heating up. It was the primary point of discussion in a recent ABC News interview with OpenAI's CEO, Sam Altman. "I'm a bit scared," he admitted, while playing up the need for carefully bringing the technology "into contact with reality."
OpenAI held back most of the details of its newest model, GPT-4, saying its previous commitment to openness "was a mistake," citing safety but also competitive concerns. (See our recent report, The Guardians, on the history of how OpenAI flipped from a non-profit open source Paladin to closed for-profit mercenary.)
Lab experiments like Alpaca show that it may be impossible to put this technology out into the world and keep it safely under wraps. AI models learn from the data sets they are given, so if you can use one, it's now been shown how you can use the results it produces to train another one to get pretty much the same results, even if you don't have the source code.
It brings to mind another battle to try to keep technology under wraps, the old digital rights management campaigns to prevent people from using Napster and BitTorrent to pillage cultural archives at will.
Business rules eventually solved the problem, making it more convenient for people to pay than steal. But no one ever solved the problem of what was called "the analog hole," meaning, if you can see or hear something, you can make an analog copy of it and there is no digital tech in the world that can stop you.
Have we just seen a version of the analog hole problem for AI? Hide the code behind an API all you want, but if you can see the results, apparently you can clone a close enough version of the model. It will be interesting to see what kinds of mitigations for-profit AI developers like OpenAI, Google, Meta and others come up with to try to defend their intellectual property, and keep their work out of the hands of bad actors.
The Stanford authors admitted to the risks of releasing their work, but defended their decision, arguing it is more important to facilitate research to help find solutions to problems like this, than keep knowledge secret. I tend to agree.
It's an important problem to think through, with massive consequences, and it is far from over. Below is a long quote from the Stanford paper detailing the team's thinking. The whole thing is worth a read.
"We believe that releasing the above assets will enable the academic community to perform controlled scientific studies on instruction-following language models, resulting in better science and ultimately new techniques to address the existing deficiencies with these models.
"At the same time, any release carries some risk. First, we recognize that releasing our training recipe reveals the feasibility of certain capabilities. On one hand, this enables more people (including bad actors) to create models that could cause harm (either intentionally or not). On the other hand, this awareness might incentivize swift defensive action, especially from the academic community, now empowered by the means to perform deeper safety research on such models. Overall, we believe that the benefits for the research community outweigh the risks of this particular release."
Create
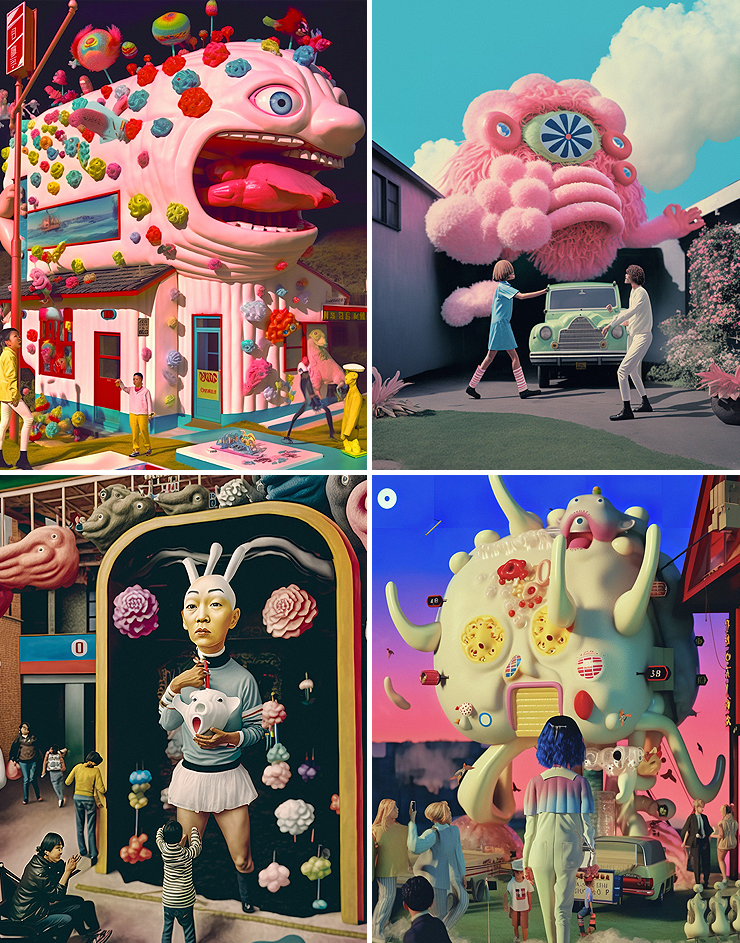
InfiniteYay's Neighborhood
Artist @infiniteyay has developed a unique and absurd style of AI art, twisting the mundane (office scenes, suburban driveways or children's bedrooms) into colorful twilight zones. His latest drop on Foundation "Neighbors" is a collection of 333 images that explore "the concept of neighbors of vastly different makeups & backgrounds existing in a wild and strange yet somehow familiar world."
RO4DM3N & H0RS3S
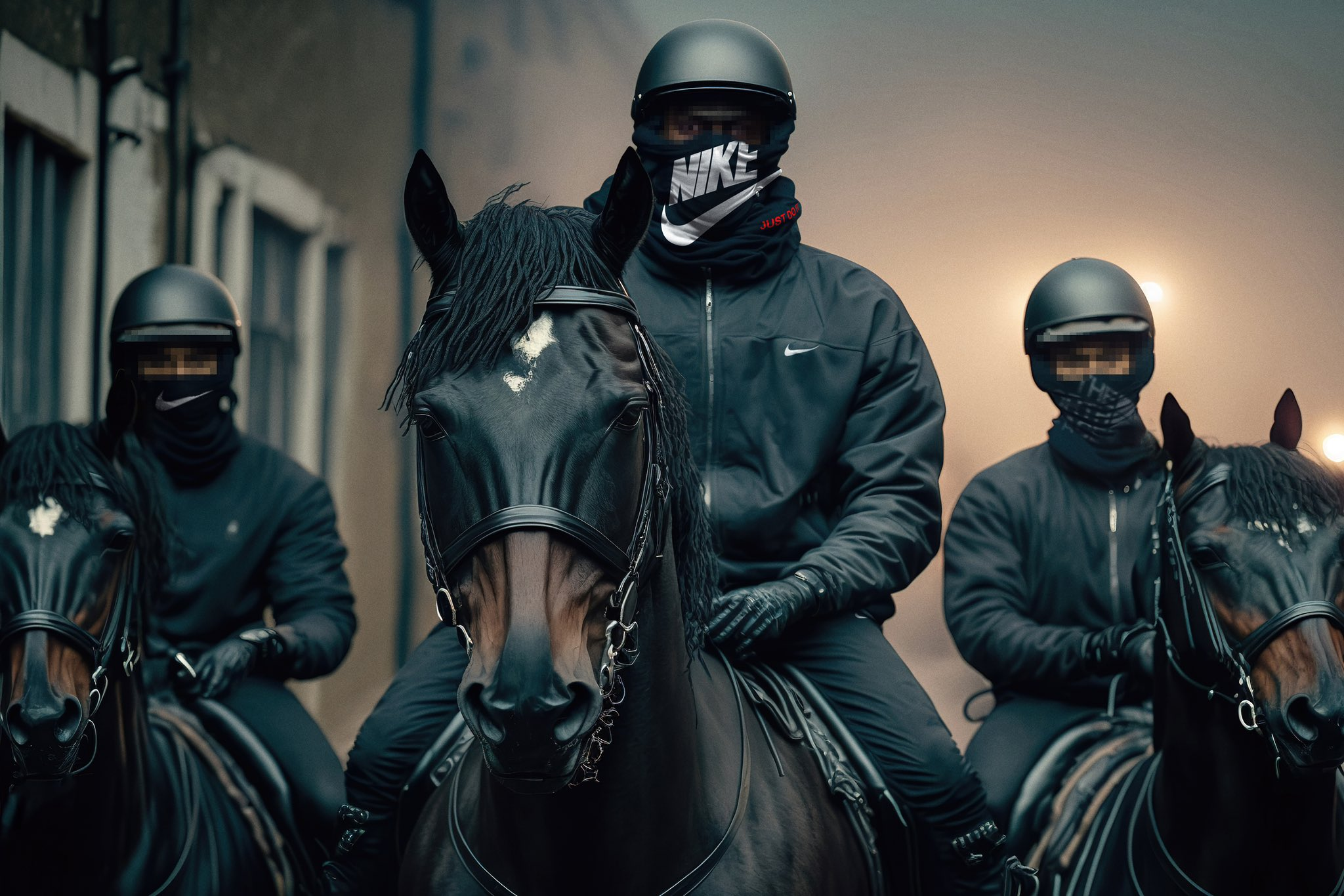
AI artist Str4ngeThing launched a new collection last week on SuperRare, 26 pieces that "uniquely imagines a world in which roadmen, iconoclasts of contemporary street fashion, flex with horses while cars don’t exist."
Compress
What's Amazon Planning with NFTs? We asked Vinay Gupta
Speculation may have ruined the familiar NFT market of PFPs and rug projects, but there are other ideas afoot that could have a more lasting impact.
Last week, Mattereum founder and CEO Vinay Gupta, a longtime leader in the blockchain field, joined our Twitter Space to explain his work around physical asset NFTs, crypto legal frameworks (not permission-less!) and shared some thoughts on Amazon's upcoming Web3 plans, and other things. Listen to the full recording to hear what a real estate NFT is and what it does (these already exist), why an Amazon NFT platform could replace Kickstarter, how real world asset NFTs can eliminate global trash, and many more provocative ideas.
Web3 Gaming Dreams Are Alive!
The Game Developers Conference is underway in San Francisco this week, and while the gaming industry has mostly taken a skeptical attitude towards Web3 some are not to be deterred. The schedule features 33 sessions focused on how crypto can super power the gaming experience, featuring talks that both point to the future and underscore the problem, including HOW TO BUILD WEB3 GAMES THAT ARE ACTUALLY FUN and HOW TO UNLOCK THE MULTI-BILLION DOLLAR ASSET TRADING MARKET WITHOUT TURNING GAMERS INTO GAMBLERS. (All Caps are the worst.)
Adept, Part I: AI Costs a Lot to Build
Fat margins traditionally scooped up by successful software companies may not flow to AI companies. Writing about Adept's recent $350 million Series B funding, Anthropic AI's Jack Clark, a member of the OpenAI breakaway team that's developing chatbot rival Claude, noted in his ImportAI newsletter that raises like this "show how frontier AI startups, though they deal in software, should be thought of as more like capital-intensive factory businesses than SaaS companies."
Adept, Part II: AI That Does More Than Chat
What is Adept? It's an AI startup that's training large-scale generative models to take multi-step actions on computers, like taking data from an Excel spreadsheet and loading it into Salesforce, all from a simple text prompt. Think of it as mainlining instruction sets instead of huffing on an LLM. Faster, more potent and likely more addictive.
Meta has released a demo of this type of utility, called Toolformer, which we wrote about a couple of weeks ago. Another game changer. (Say, did you notice Meta is dropping a lot of dope AI lately?)
AI In the UK
The political implications of artificial general intelligence are starting to get noticed. This policy recommendation paper, originally written privately for the UK government, is a bit wonky but makes some great points that could be applied everywhere, and is highly relevant to the open vs closed AI debate above. The author says UK academics in charge of driving the country's AI strategy missed the boat on neural networks, the special sauce in OpenAI's products, costing Britain a decade in publicly owned AI research. A more entrepreneurial mindset is needed. Why would anyone want to cede control of this technology to a few private venture-backed American companies? Good question.
Cool Tools
RunwayML Gen2
Making video from text prompts just got a whole lot better.
The Verge: We're Impressed!
From Clippy to Co-Pilot, Microsoft finally nails the digital assistant.
Reimagine an Image
Stability AI has unveiled ClipDrop, a tool that introduces small or large changes into an existing image - like a new furniture arrangement from a photo of your living room.
ChatGPT for Game Design
Write a text prompt, get a virtual environment in Unity's Game Engine.
That Was Fast
Free access to GPT-4 with Replit is now waitlisted.